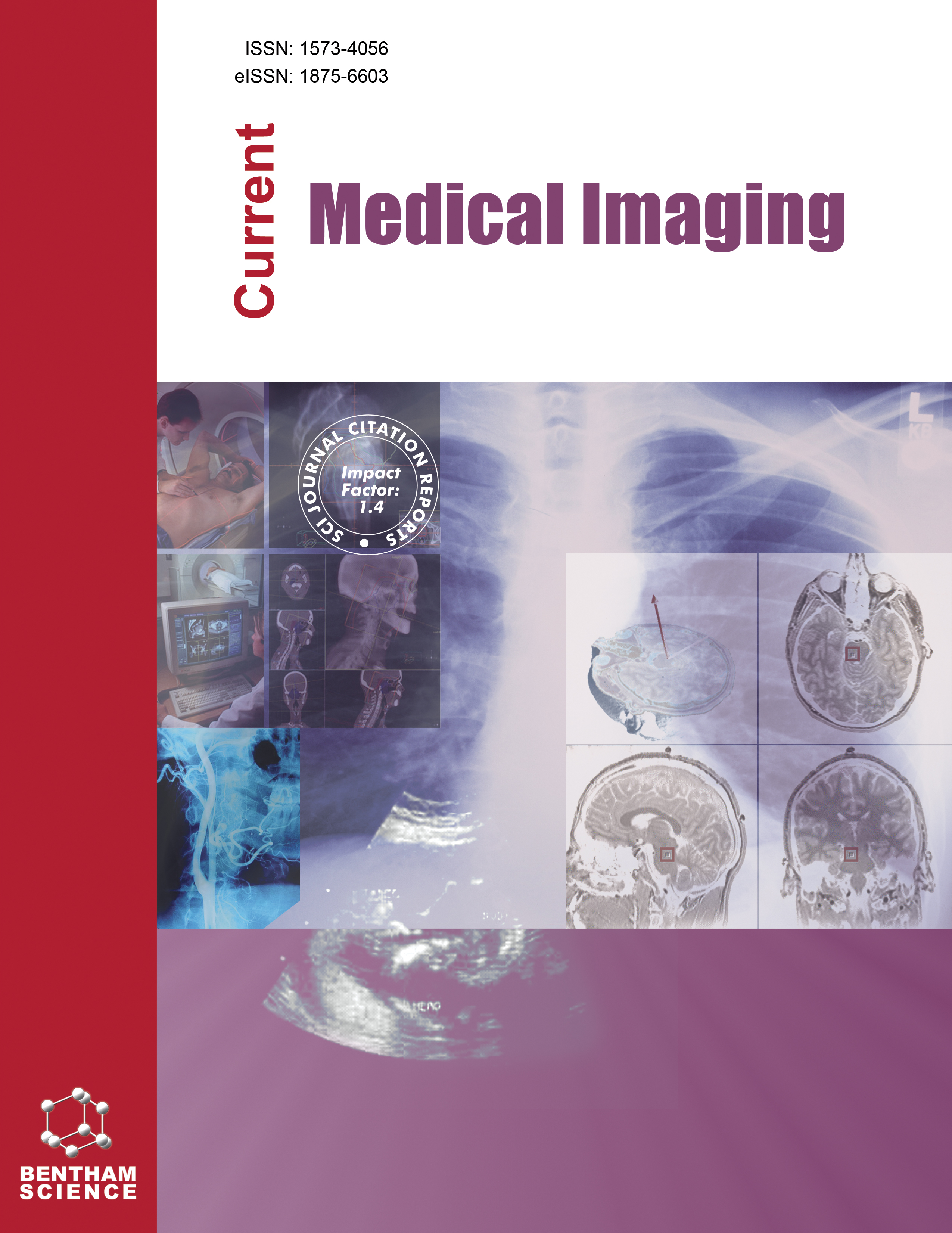
-
oa Differentiation of Minute Pulmonary Meningothelial-Like Nodules and Adenocarcinoma In situ with CT Radiomics
-
-
- 01 Sep 2024
- 02 Jan 2025
- 14 Mar 2025
Abstract
An effective preoperative diagnosis between minute pulmonary meningothelial-like nodules (MPMNs) and adenocarcinoma in situ (AIS) can provide clinicians with appropriate treatment strategies.
This study aimed to differentiate MPMNs from AIS via computed tomography (CT) radiomics approaches.
Clinical and imaging data from fifty-one patients diagnosed with MPMNs and 55 patients diagnosed with AIS were collected from Jiangsu Province Hospital and Nanjing First Hospital from January 2016 to December 2022. All patients underwent chest CT scans before surgery. All CT images were segmented with ITK-SNAP software, and the radiomics features were further extracted with the Python PyRadiomics package. Least absolute shrinkage and selection operator (LASSO) regression analysis was used to select the optimal radiomics features for the construction of the model. The ROC curve was used to evaluate the diagnostic efficacy of the model.
After feature reduction and selection, 16 radiomics features were selected to construct the radiomics model. In the test set, the sensitivity, specificity, positive predictive value, negative predictive value, and accuracy of the k-nearest neighbor model were 87.5%, 88.9%, 96.9%, 77.8%, and 88.5%, respectively, and the AUC of the ROC curve was 0.969 (95% CI: 0.72-1.00).
The CT radiomics model has exhibited high diagnostic value in the differential diagnosis between MPMNs and AIS.