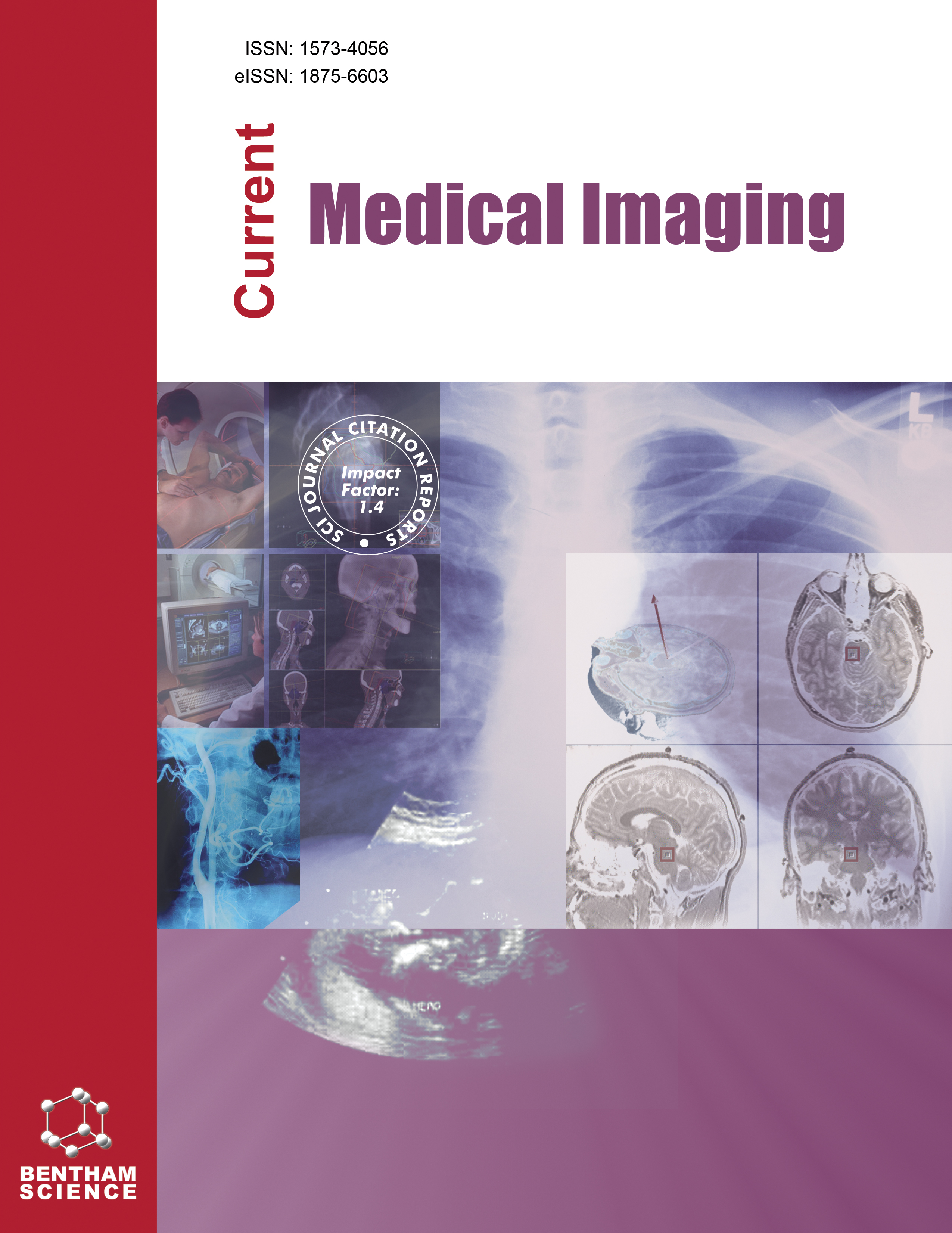
-
oa Personalized Respiratory Motion Modeling Incorporating Longitudinal Data through Two-stage Transfer Learning
-
-
- 16 May 2024
- 20 Nov 2024
- 21 Jan 2025
Abstract
This study aims to develop an accurate image registration framework for personalized respiratory motion modeling.
The proposed framework incorporates longitudinal data through a two-stage transfer learning approach. In the first stage, transfer learning is employed on longitudinal data collected from the same device. In the second stage, a personalized model is constructed using the transfer learning approach, reusing the model from the first stage. A novel cross-error function is introduced to guide the customized adaptation stage.
The experiments demonstrate the effectiveness of the proposed framework in respiratory motion modeling. Integrating longitudinal data allows for improved accuracy for personalized respiratory motion modeling.
The study presents a novel approach that incorporates longitudinal data into a two-stage transfer learning process for personalized respiratory motion modeling. The framework demonstrates improved accuracy. The results highlight the potential of leveraging longitudinal data to provide personalized image registration solutions.