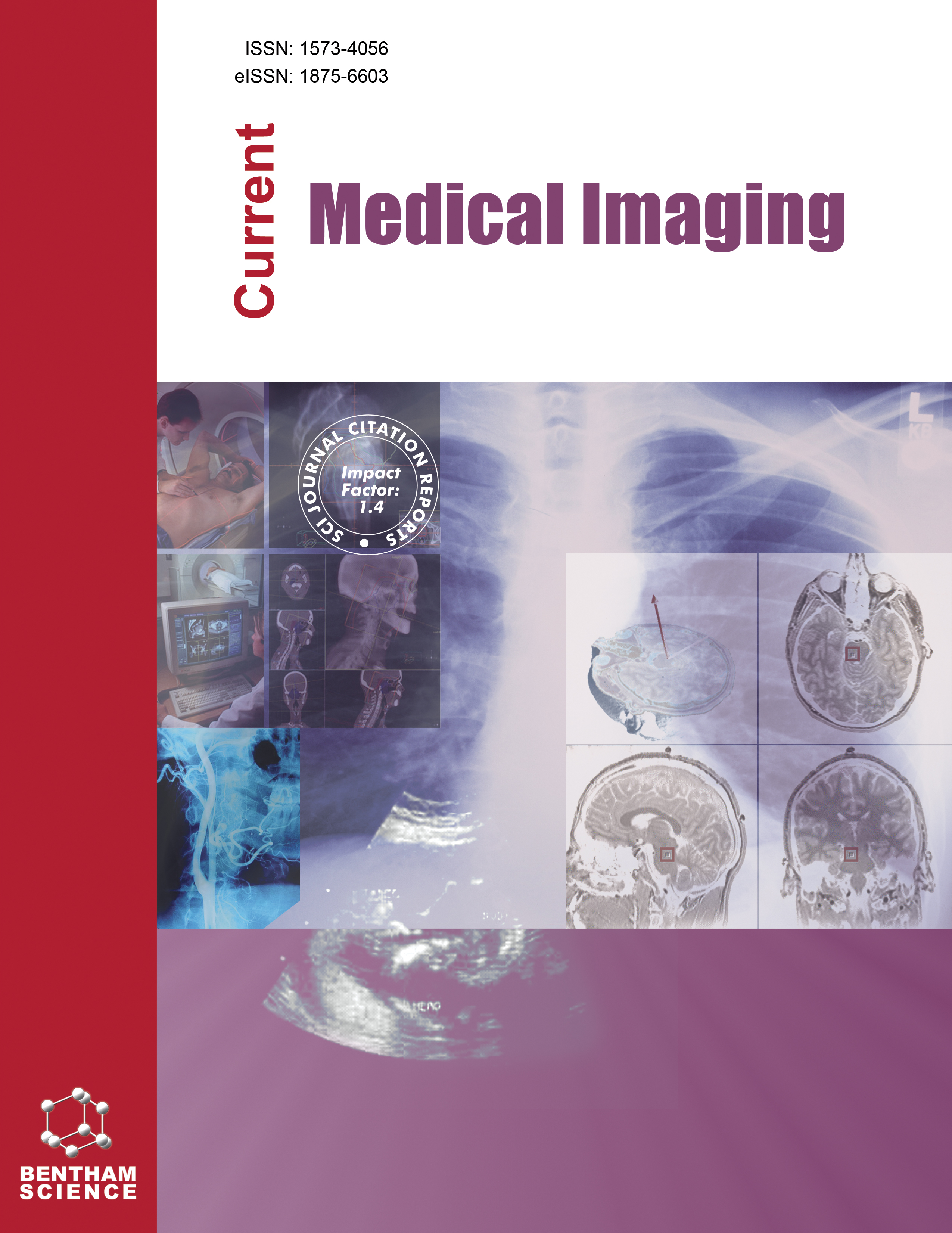
-
oa CERVIXNET: An Efficient Approach for the Detection and Classifications of the Cervigram Images Using Modified Deep Learning Architecture
-
-
- 05 Aug 2024
- 29 Nov 2024
- 23 Jan 2025
Abstract
The earlier detection of cervical cancer in women patients can save human life. This article proposes a novel methodology for detecting abnormal cervigram images from healthy cervigram images and segments the cancer regions in the abnormal cervigram images using the deep learning method. The conventional deep learning architecture has been modified into the proposed CervixNet architecture to improve the cervical cancer detection rate.
This methodology is constituted of a training and testing process, where the training process generates the training sequences individually for healthy cervigram images and the cancer case cervigram images. The testing process tests the cervigram images into either a healthy or cancer cases using the training sequences generated through the training process. During the testing process of the proposed system, the cancer segmentation algorithm was applied on the abnormal cervigram image to detect and segment the pixels belonging to cancer. Finally, the performance has been carried out on the segmented cancer cervical images for the ground truth images. This proposed methodology has been evaluated on the cervigrams on IMODT and Guanacaste databases. Its performance has been analyzed concerning cancer pixel sensitivity, cancer pixel specificity and cancer pixel accuracy.
This research work obtains 98.69% Cancer Pixel Sensitivity (CPS), 98.76% Cancer Pixel Specificity (CPSP), and 99.27% Cancer Pixel Accuracy (CPA) for the set of cervigram images in the IMODT database. This research work obtains 99.22% CPS, 99.03% CPSP, and 99.01% CPA for the set of cervigram images in Guanacaste database.
These experimental results of the proposed work have been significantly compared with the state-of-the-art methods and show the significance and novelty of the proposed works.