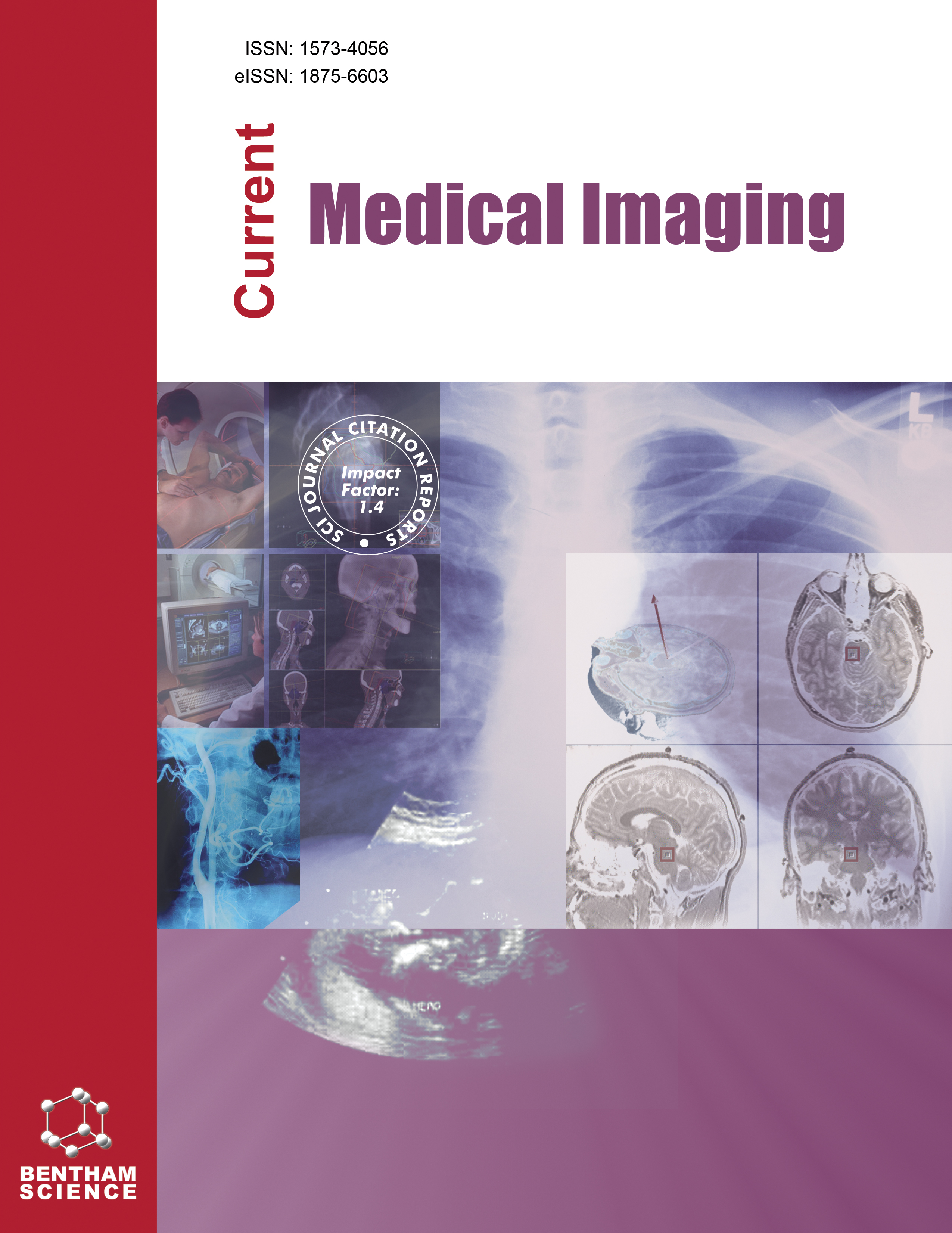
-
oa Deep Learning-assisted Diagnosis of Extrahepatic Common Bile Duct Obstruction Using MRCP Imaging and Clinical Parameters
-
-
- 11 Oct 2024
- 21 Nov 2024
- 20 Jan 2025
Abstract
Extrahepatic Common Bile Duct Obstruction (EHBDO) is a serious condition that requires accurate diagnosis for effective treatment. Magnetic Resonance Cholangiopancreatography (MRCP) is a widely used noninvasive imaging technique for visualizing bile ducts, but its interpretation can be complex.
This study aimed to develop a deep learning-based classification model that integrates MRCP images and clinical parameters to assist radiologists in diagnosing EHBDO more accurately.
A total of 465 patients with clinical data were included, of whom 143 also had MRCP images. Missing clinical values were addressed through data imputation. Object detection techniques were used to isolate the common bile duct region in the MRCP images. A multimodal deep learning fusion model was developed by combining the extracted imaging features with selected clinical parameters. To account for the varying significance of different features, a weighted loss function was applied. The performance of the fusion model was compared to that of single-modality approaches (using only MRCP images or clinical data), specifically the accuracy, sensitivity, specificity, and Area Under The Curve (AUC).
The performance of the proposed deep learning fusion model was superior to that of models using only MRCP images or clinical parameters. The fusion model achieved an accuracy of 89.8%, AUC of 90.4%, sensitivity of 81.8%, and specificity of 95.7% in diagnosing EHBDO. By integrating MRCP imaging data and clinical parameters, the proposed deep learning model significantly enhanced the accuracy of EHBDO diagnosis.
This proposed multimodal approach outperformed traditional single-modality methods, presenting a valuable tool for improving the diagnostic accuracy of bile duct obstruction.