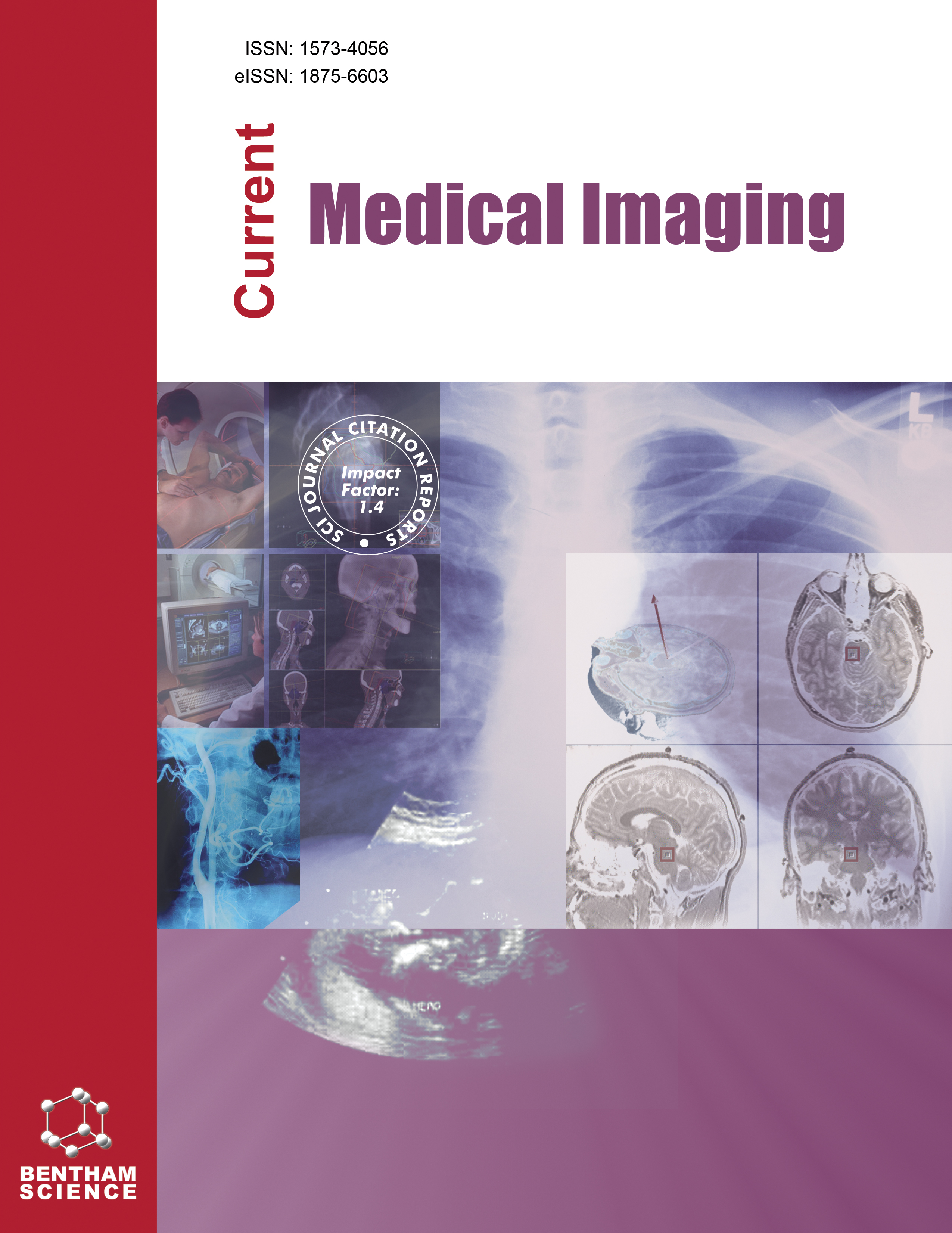
-
oa Advanced CNN Architecture for Brain Tumor Segmentation and Classification using BraTS-GOAT 2024 Dataset
-
-
- 09 Aug 2024
- 13 Nov 2024
- 02 Jan 2025
Abstract
The BraTS Generalizability Across Tumors (BraTS-GoAT) initiative addresses the critical need for robust and generalizable models in brain tumor segmentation. Despite advancements in automated segmentation techniques, the variability in tumor characteristics and imaging modalities across clinical settings presents a significant challenge.
This study aims to develop an advanced CNN-based model for brain tumor segmentation that enhances consistency and utility across diverse clinical environments. The objective is to improve the generalizability of CNN models by applying them to large-scale datasets and integrating robust preprocessing techniques.
The proposed approach involves the application of advanced CNN models to the BraTS 2024 challenge dataset, incorporating preprocessing techniques such as standardization, feature extraction, and segmentation. The model's performance was evaluated based on accuracy, mean Intersection over Union (IOU), average Dice coefficient, Hausdorff 95 score, precision, sensitivity, and specificity.
The model achieved an accuracy of 98.47%, a mean IOU of 0.8185, an average Dice coefficient of 0.7, an average Hausdorff 95 score of 1.66, a precision of 98.55%, a sensitivity of 98.40%, and a specificity of 99.52%. These results demonstrate a significant improvement over the current gold standard in brain tumor segmentation.
The findings of this study contribute to establishing benchmarks for generalizability in medical imaging, promoting the adoption of CNN-based brain tumor segmentation models in diverse clinical environments. This work has the potential to improve outcomes for patients with brain tumors by enhancing the reliability and effectiveness of automated segmentation techniques.