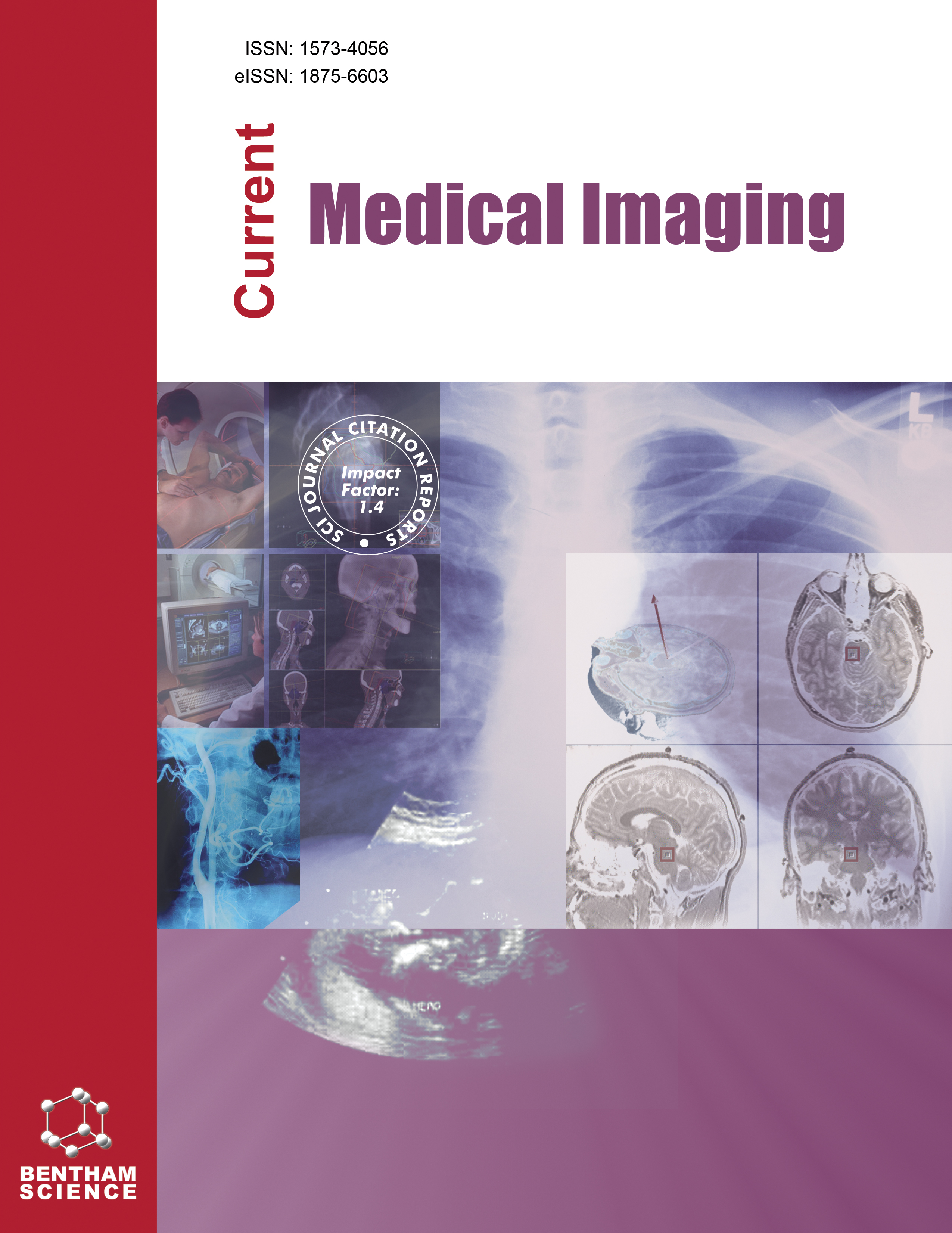
-
oa Lightweight Lung-nodule Detection Model Combined with Multidimensional Attention Convolution
-
-
- 12 May 2024
- 06 Nov 2024
- 02 Jan 2025
Abstract
Early and timely detection of pulmonary nodules and initiation treatment can substantially improve the survival rate of lung carcinoma. However, current detection methods based on convolutional neural networks (CNNs) cannot easily detect pulmonary nodules owing to low detection accuracy and the difficulty in detecting small-sized pulmonary nodules; meanwhile, more accurate CNN-based models are slow and require high hardware specifications.
The aim of this study is to develop a detection model that achieves both high accuracy and real-time performance, ensuring effective and timely results.
In this study, based on YOLOv5s, a concentrated-comprehensive convolution (C3_ODC) module with multidimensional attention is designed in the convolutional layer of the original backbone network for enhancing the feature-extraction capabilities of the model. Moreover, lightweight convolution is combined with weighted bidirectional feature pyramid networks (BiFPNs) to form a GS-BiFPN structure that enhances the fusion of multiscale features while reducing the number of model parameters. Finally, Focal Loss is combined with the normalized Wasserstein distance (NWD) to optimize the loss function. Focal loss focuses on carcinoma-positive samples to mitigate class imbalance, whereas the NWD enhances the detection performance of small lung nodules.
In comparison experiments against the YOLOv5s, the proposed model improved the average precision by 8.7% and reduced the number of parameters and floating-point operations by 5.4% and 8.2%, respectively, while achieving 116.7 frames per second.
The proposed model balances high detection accuracy against real-time requirements.