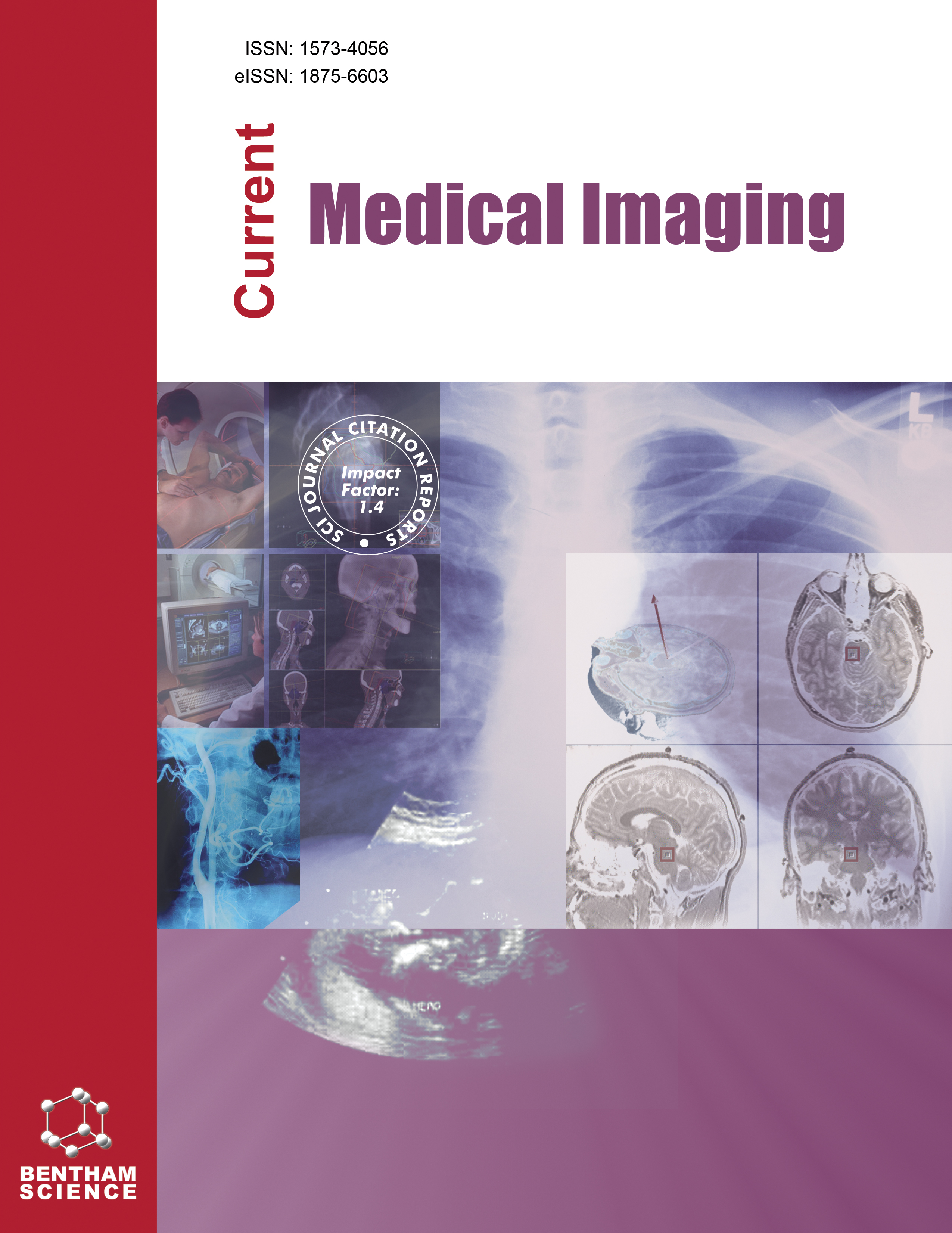
-
oa Machine-Learning Based Computed Tomography Radiomics Nomgram For Predicting Perineural Invasion In Gastric Cancer
-
-
- 19 May 2024
- 27 Nov 2024
- 13 Jan 2025
Abstract
The aim of this study was to develop and validate predictive models for perineural invasion (PNI) in gastric cancer (GC) using clinical factors and radiomics features derived from contrast-enhanced computed tomography (CE-CT) scans and to compare the performance of these models.
This study included 205 GC patients, who were randomly divided into a training set (n=143) and a validation set (n=62) in a 7:3 ratio. Optimal radiomics features were selected using the least absolute shrinkage and selection operator (LASSO) algorithm. A radiomics model was constructed utilizing the optimal among five machine learning filters, and a radiomics score (rad-score) was computed for each participant. A clinical model was built based on clinical factors identified through multivariate logistic regression. Independent clinical factors were combined with the rad-score to create a combined radiomics nomogram. The discrimination ability of the models was evaluated by receiver operating characteristic (ROC) curves and the DeLong test.
Independent predictive factors of the clinical model included tumor T stage, N stage, and tumor differentiation, with AUC values of 0.777 and 0.809 in the training and validation sets. The radiomics model was constructed using the support vector machine (SVM) classifier with the best AUC (0.875 in the training set and 0.826 in the validation set). The combined radiomics nomogram, which combines independent clinical predictors and the rad-score, demonstrated better predictive performance (AUC=0.889 in the training set; AUC=0.885 in the validation set).
The nomogram integrating independent clinical predictors and CE-CT radiomics was constructed to predict PNI in GC. This model demonstrated favorable performance and could potentially assist in prognosis evaluation and clinical decision-making for GC patients.