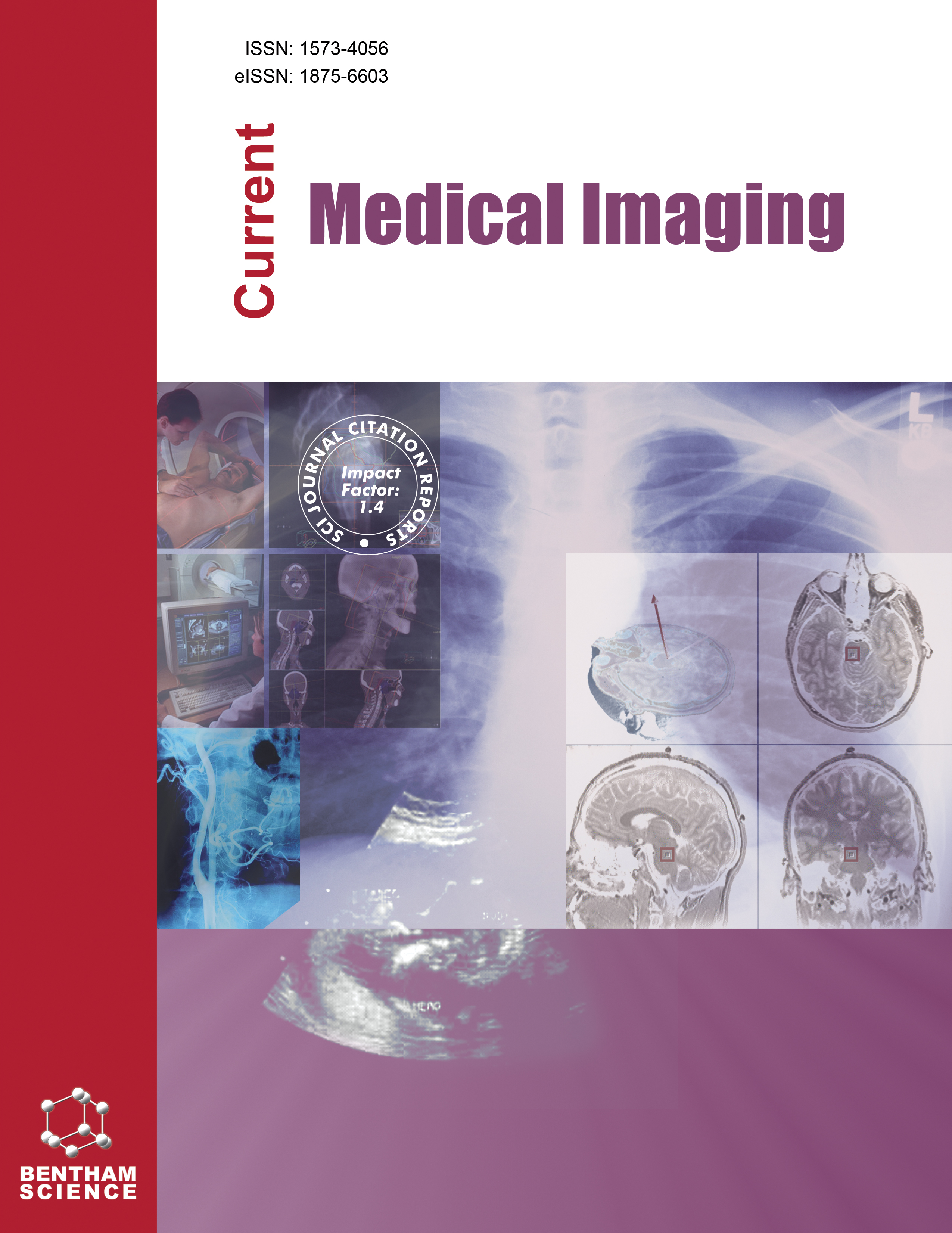
-
oa YOLOv8 Algorithm-aided Detection of Rib Fracture on Multiplane Reconstruction Images
-
-
- 14 Jun 2024
- 06 Dec 2024
- 17 Mar 2025
Abstract
This study aimed to develop and assess the performance of a YOLOv8 algorithm-aided detection model for identifying rib fractures on multiplane reconstruction (MPR) images, addressing the limitations of current AI models and the labor-intensive nature of manual diagnosis.
Ethical approval was obtained, and a dataset comprising 624 MPR images, confirmed by CT, was collected from three regions of Tongji Hospital between May 2020 and May 2023. The images were categorized into training, validation, and external test sets. A musculoskeletal radiologist labeled the images, and a YOLOV8n model was trained and validated using these datasets. The performance metrics, including sensitivity, specificity, accuracy, precision, recall, and F1 score, were calculated.
The refined YOLO model demonstrated high diagnostic accuracy, with sensitivity, specificity, and accuracy rates of 96%, 97%, and 97%, respectively. The AI model significantly outperformed the radiologist in terms of diagnostic speed, with an average interpretation time of 2.02 seconds for 144 images compared to 288 seconds required by the radiologist.
The YOLOv8 algorithm shows promise in expediting the diagnosis of rib fractures on MPR images with high accuracy, potentially improving clinical efficiency and reducing the workload for radiologists. Future work will focus on enhancing the model with more feature learning capabilities and integrating it into the PACS system for human-computer interaction.