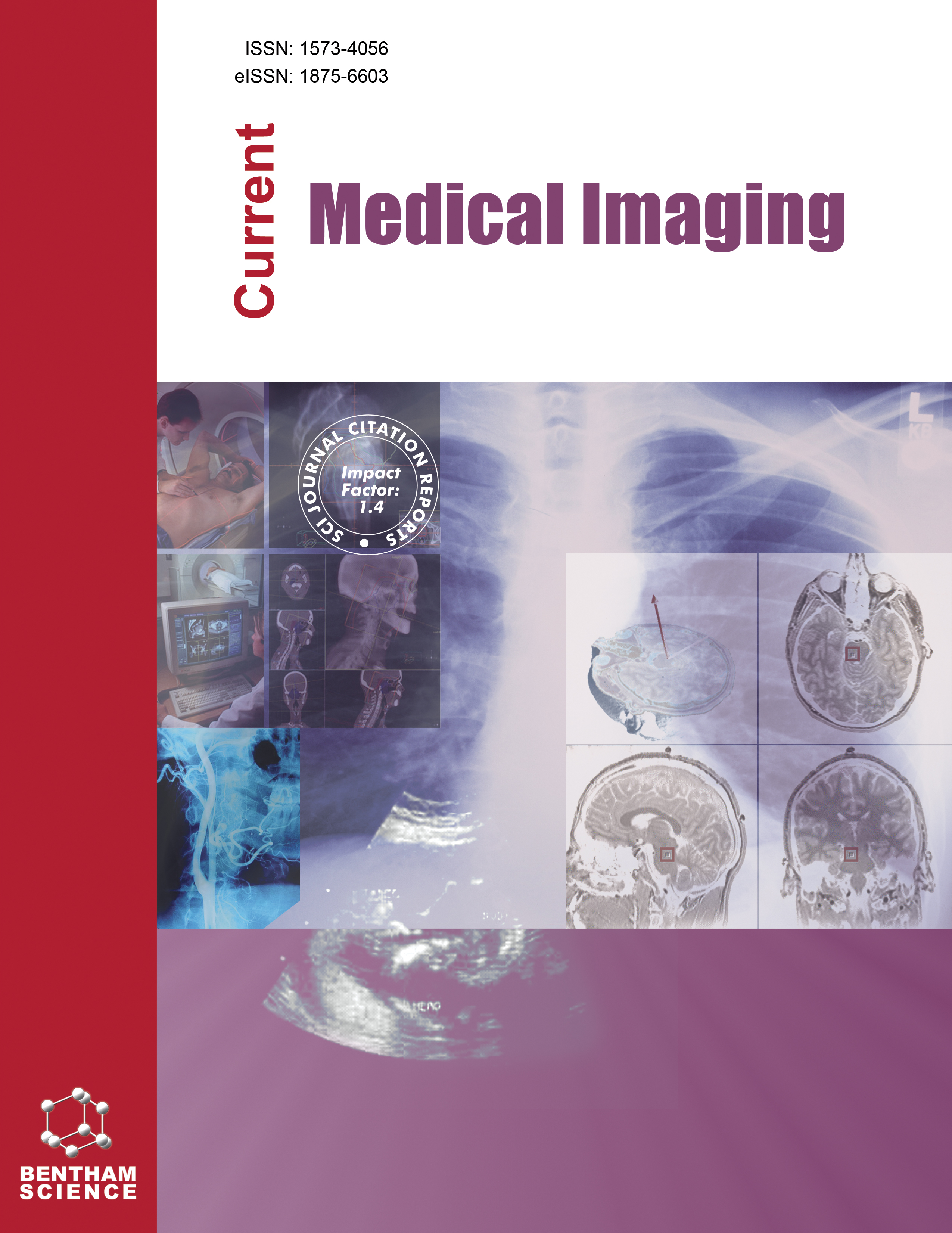
-
oa Enhanced Pneumonia Detection in Chest X-Rays Using Hybrid Convolutional and Vision Transformer Networks
-
-
- 24 Jun 2024
- 20 Nov 2024
- 09 Jan 2025
Abstract
The objective of this research is to enhance pneumonia detection in chest X-rays by leveraging a novel hybrid deep learning model that combines Convolutional Neural Networks (CNNs) with modified Swin Transformer blocks. This study aims to significantly improve diagnostic accuracy, reduce misclassifications, and provide a robust, deployable solution for underdeveloped regions where access to conventional diagnostics and treatment is limited.
The study developed a hybrid model architecture integrating CNNs with modified Swin Transformer blocks to work seamlessly within the same model. The CNN layers perform initial feature extraction, capturing local patterns within the images. At the same time, the modified Swin Transformer blocks handle long-range dependencies and global context through window-based self-attention mechanisms. Preprocessing steps included resizing images to 224x224 pixels and applying Contrast Limited Adaptive Histogram Equalization (CLAHE) to enhance image features. Data augmentation techniques, such as horizontal flipping, rotation, and zooming, were utilized to prevent overfitting and ensure model robustness. Hyperparameter optimization was conducted using Optuna, employing Bayesian optimization (Tree-structured Parzen Estimator) to fine-tune key parameters of both the CNN and Swin Transformer components, ensuring optimal model performance.
The proposed hybrid model was trained and validated on a dataset provided by the Guangzhou Women and Children’s Medical Center. The model achieved an overall accuracy of 98.72% and a loss of 0.064 on an unseen dataset, significantly outperforming a baseline CNN model. Detailed performance metrics indicated a precision of 0.9738 for the normal class and 1.0000 for the pneumonia class, with an overall F1-score of 0.9872. The hybrid model consistently outperformed the CNN model across all performance metrics, demonstrating higher accuracy, precision, recall, and F1-score. Confusion matrices revealed high sensitivity and specificity with minimal misclassifications.
The proposed hybrid CNN-ViT model, which integrates modified Swin Transformer blocks within the CNN architecture, provides a significant advancement in pneumonia detection by effectively capturing both local and global features within chest X-ray images. The modifications to the Swin Transformer blocks enable them to work seamlessly with the CNN layers, enhancing the model’s ability to understand complex visual patterns and dependencies. This results in superior classification performance. The lightweight design of the model eliminates the need for extensive hardware, facilitating easy deployment in resource-constrained settings. This innovative approach not only improves pneumonia diagnosis but also has the potential to enhance patient outcomes and support healthcare providers in underdeveloped regions. Future research will focus on further refining the model architecture, incorporating more advanced image processing techniques, and exploring explainable AI methods to provide deeper insights into the model's decision-making process.