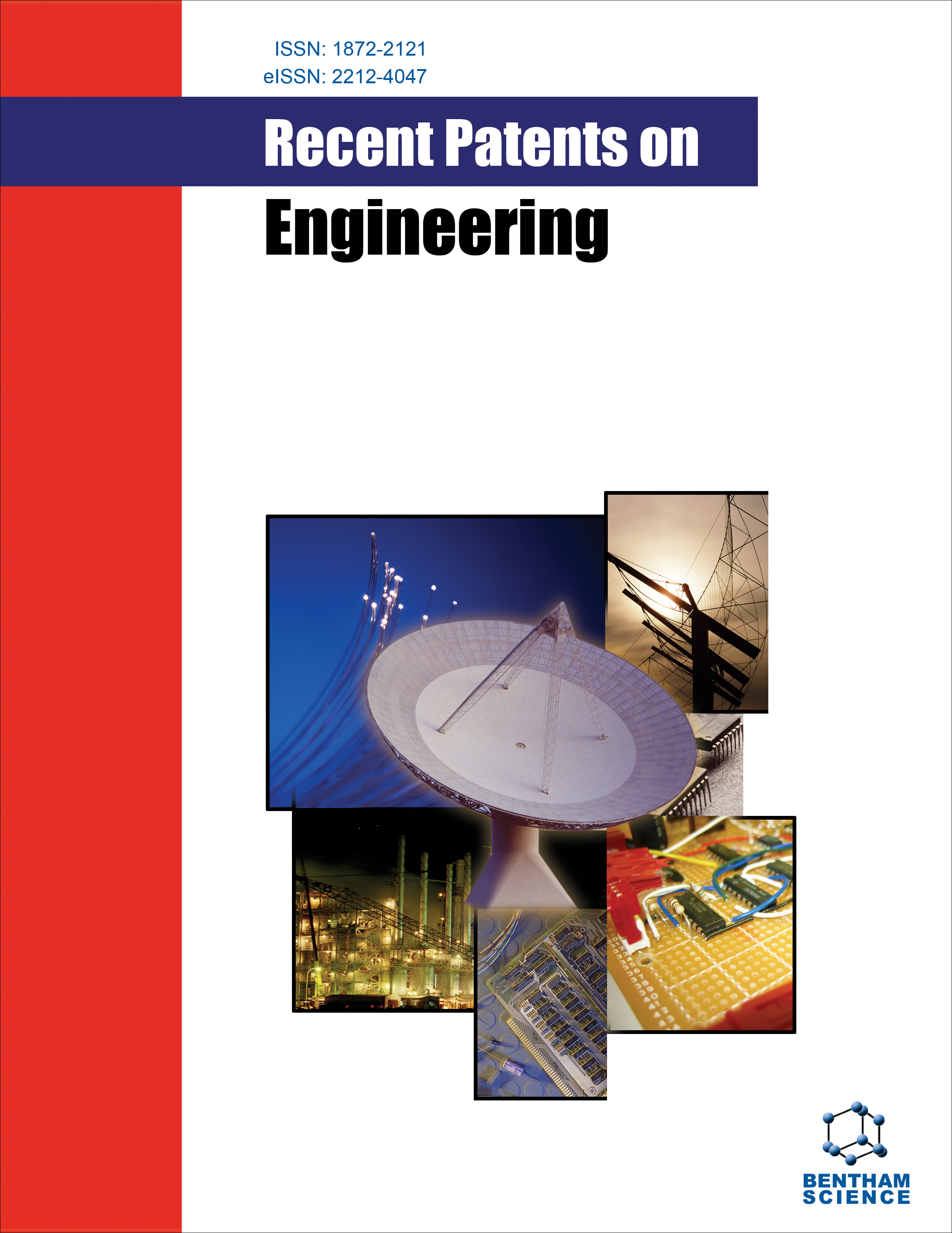
-
oa Identification and Control of Transportation Units with Sequenced Learning Models Using Neural Networks
-
-
- 23 Jun 2024
- 16 Sep 2024
- 09 Dec 2024
Abstract
This study illustrates the significance of transport units in monitoring diverse paths using a critical system model. The suggested method identifies proficiency and framework patterns that evolve across different time intervals, utilising machine learning optimisation that incorporates sequence learning with interconnected neural networks.
As an increasing number of cars are interconnected for data communication to illustrate available routes, it is essential to have suitable connectivity for transportation units. This study may facilitate intelligent connectivity across transportation units by employing essential shifts without compromising the efficiency of connected units.
This study aimed to integrate the parametric design representations with neural networks to address the primary goal of min-max functions, hence enhancing the efficiency of transportation units.
The method presented here has employed sequenced learning patterns to select the shortest path while rapidly altering pathway representations.
The alterations in pathways influenced by emissions have been noted and excluded from connectivity units to enhance the overall lifetime of transportation units in the projected model.
The results have been examined through a simulation framework encompassing four scenarios, wherein potential connectedness has enhanced both the proficiency rate and the structure while minimising the shifts. Subsequently, a comparison of the proposed method with the existing methodology, where total efficiency has been assessed, has revealed the proposed method to maximise the efficiency to 95%. In contrast, the existing strategy has yielded a reduced efficiency of 86%.