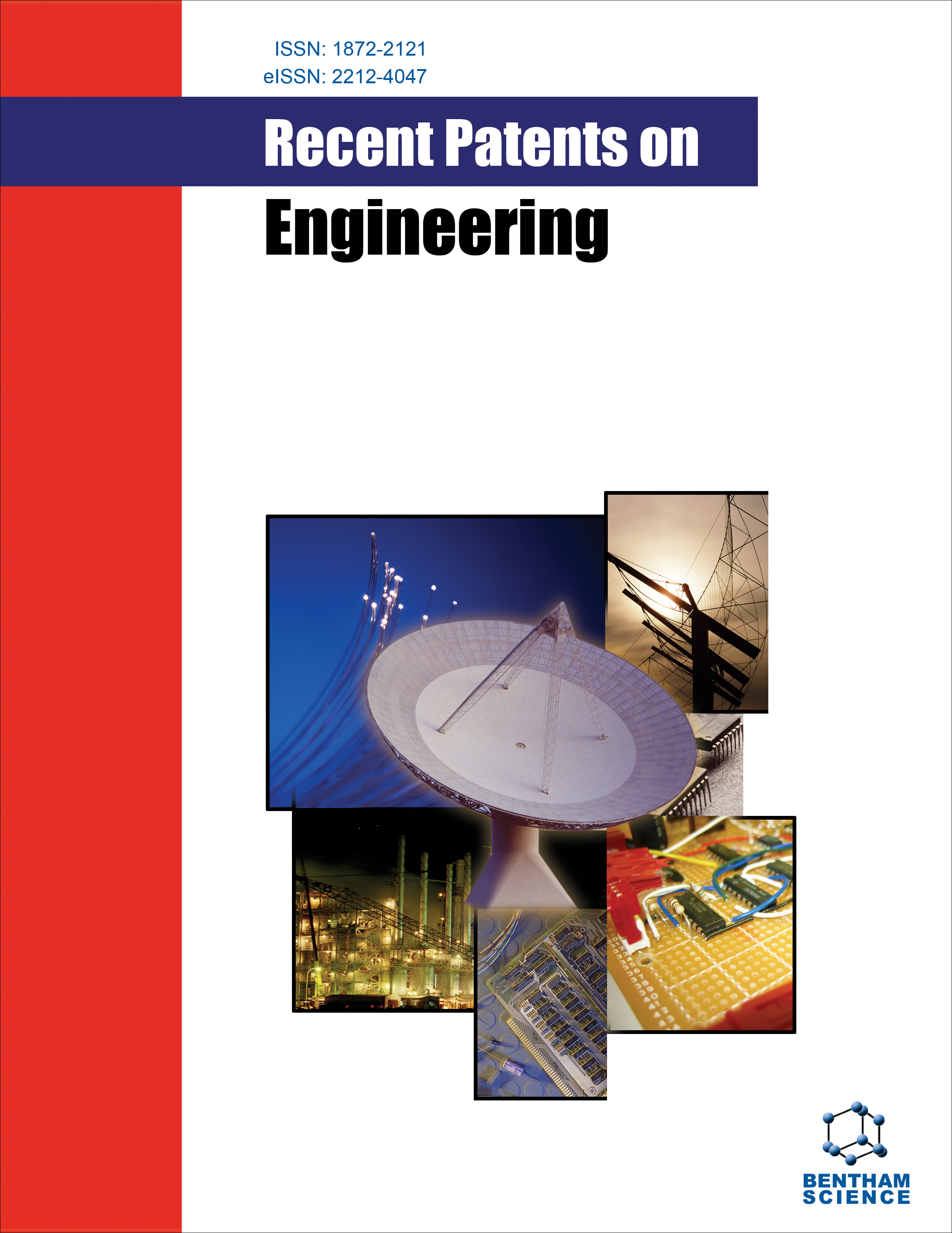
-
oa Hofstede’s Cultural Dimension Driven Artificial Narrow Intelligence iDFIS for Industry 5.0 Empowered Digital Society
-
-
- 18 Jun 2024
- 29 Aug 2024
- 19 Dec 2024
- Previous Article
- Table of Contents
- Next Article
Abstract
The fields which are considered to be most primary adopters of AI are financial services, banking and insurance sectors. The implementations of AI technology patent are mostly in the fields of investments, securities, consumer relationships, risk management, market analysis and compliance. AI of today is a collection of lots of algorithms and techniques that are good for variety of applications. Another variant of AI patent that is Artificial Narrow Intelligence (ANI) can perform task which is specific or can perform narrow range of task.
ANI models are intelligent enough to perform well define task within a limited domain.
ANI model operates within the well-defined parameters. The current study aims to discuss the customers view on adoption of an ANI enabled autonomous intelligent digital financial inclusion system (iDFIS) patent.
Total 681 responses were collected from different state of India. The result shows that the factor like convenience, performance efficiency, security, personalization, effort efficiency and social influence factor had a significant positive relationship with the attitude to adopt an autonomous iDFIS system. The three cultural dimension of Hofstede’s theory power distance index, collectivism and uncertainty acceptance also taken as a moderator. The effect of moderators also seen in the different relationships.
The finding of the study will be worthy to develop a strategic decision making in finance sector. The goal of finance sector is also focus on new AI innovative technologies to improve overall growth related to all aspect of finance sector specially in the field of customer service.
The study proposed the direct hypotheses to analyze the adoption of an autonomous iDFIS system among customers.