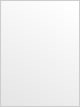
-
oa A Machine Learning-Based Multi-Model Approach for Predicting Post-Deep Brain Stimulation Outcomes in Alzheimer’s disease Patients
-
-
- 29 Jul 2024
- 21 Oct 2024
- 10 Dec 2024
Abstract
Deep-brain stimulation (DBS) is a demonstrated rehabilitation for Alzheimer's Disease (AD), regularly resulting in an upgrade of engine work. In any case, some unfortunate incidental upshots can occur past DBS, which can deteriorate the patient's satisfaction. In this way, the medical group must painstakingly choose patients over whom to perform DBS. In the past, there have been a few endeavors to associate pre-usable information and DBS experimental results, generally centered on engine symptomatology.
A machine learning (ML)-based strategy called a multimodel pipeline alluded to as FlowModel is designed and implemented to anticipate an enormous number of DBS medical results for AD. It is composed of a patented Artificial Neural Network (ANN) for processing medical data, using a conventional image handling technique to extract morphological biomarkers from T1 imaging, and employing a Support Vector Machine (SVM) to perform regressions. We authenticated it in 256 AD patients who underwent DBS.
The FlowModel demonstrated high predictive precision, achieving a correlation coefficient of as high as 0.82 and successfully predicting 63 out of 84 clinical outcome scores. Importantly, FlowModel outperformed traditional linear models, demonstrating the capability to learn effectively from preoperative data alone.
The uniqueness of this study lies in its ability to predict multiple clinical outcomes across different modalities, independent of stimulation parameters. The introduction of this ML pipeline contributes to the precision medicine landscape by providing a robust, data-driven tool to select patients for DBS, potentially improving clinical decision-making and patient outcomes.