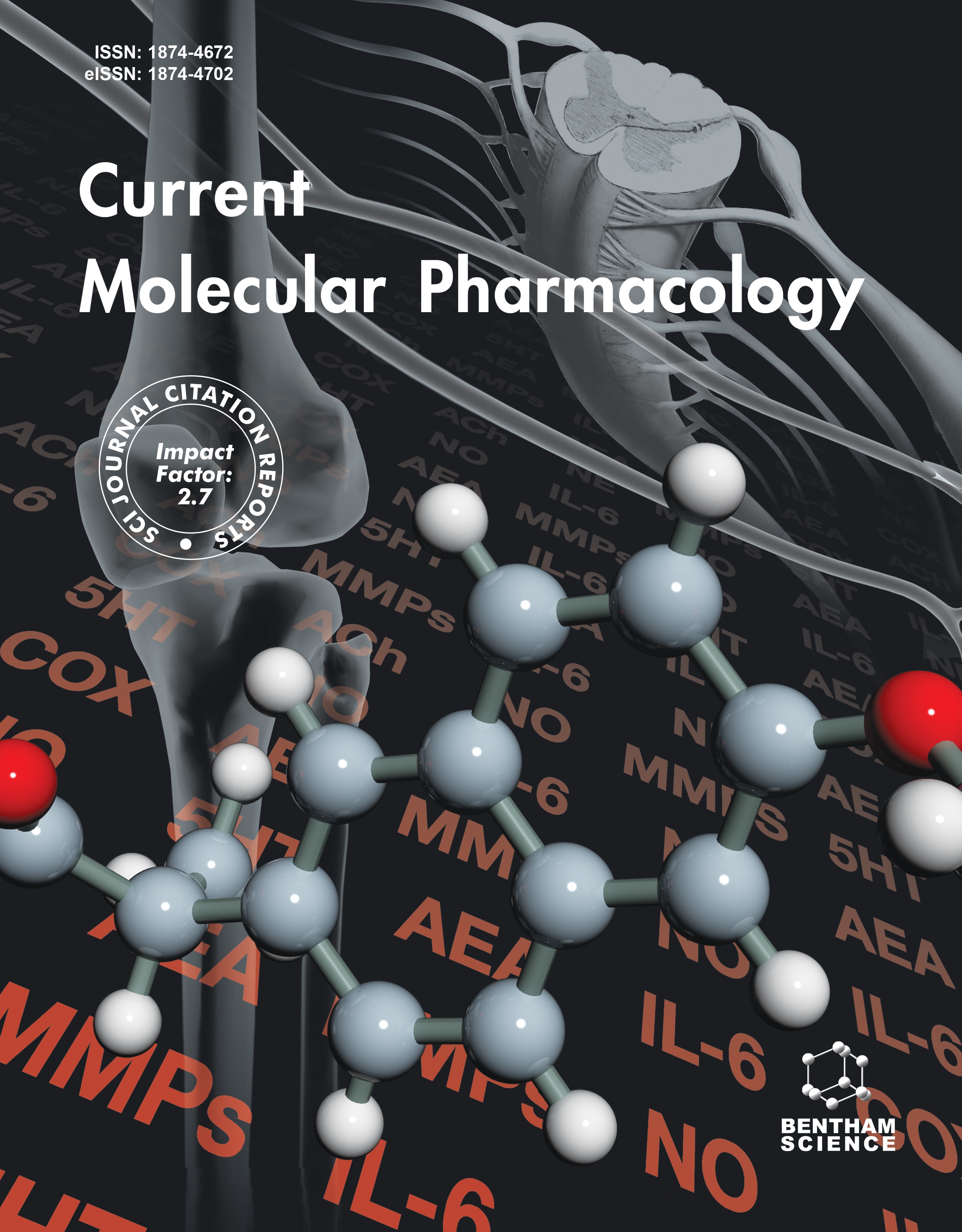
-
oa Exploring the Immune-Related Molecular Mechanisms Underlying the Comorbidity of Temporal Lobe Epilepsy and Major Depressive Disorder through Integrated Data Set Analysis
-
-
- 26 Nov 2024
- 22 Jan 2025
- 18 Feb 2025
- Previous Article
- Table of Contents
- Next Article
Abstract
Temporal lobe epilepsy (TLE) and major depressive disorder (MDD) are prevalent and complex neurological disorders that affect individuals globally. Clinical and epidemiological studies indicate a significant comorbidity between TLE and MDD; however, the shared molecular mechanisms underlying this relationship remain unclear. This study aims to explore the common key genes associated with TLE and MDD through a systematic analysis of gene expression profiles, elucidate their underlying molecular pathological mechanisms, and evaluate the potential applications of these genes in diagnostic and therapeutic contexts.
Brain tissue gene expression data for TLE and MDD were obtained from the GEO database. Differentially expressed genes (DEGs), weighted gene co-expression network analysis (WGCNA), functional enrichment, and protein-protein interaction (PPI) network construction were performed to identify shared gene modules. LASSO and random forest (RF) machine learning models were used to select diagnostic candidate genes, validated through ROC curve analysis. Immune infiltration analysis explored the immune involvement of key genes, while single-cell sequencing confirmed gene expression across cell types. Potential therapeutic drugs were identified using a drug database.
A total of 372 DEGs were identified as either up- or down-regulated between TLE and MDD, with WGCNA revealing nine shared gene modules. Seven hub genes, including HTR7 and CDHR2, demonstrated strong ROC performance. Immune infiltration analysis revealed changes in immune cell populations linked to key genes, confirmed by single-cell sequencing. Upadacitinib was identified as a potential therapeutic drug targeting these genes.
This study identified shared gene expression profiles between TLE and MDD, emphasizing immune pathway-related molecular mechanisms. Immune infiltration analysis and single-cell sequencing underscored the significance of immune regulation in their comorbidity, while drug prediction highlights candidates for precision medicine, establishing a foundation for future research and therapeutic strategies.