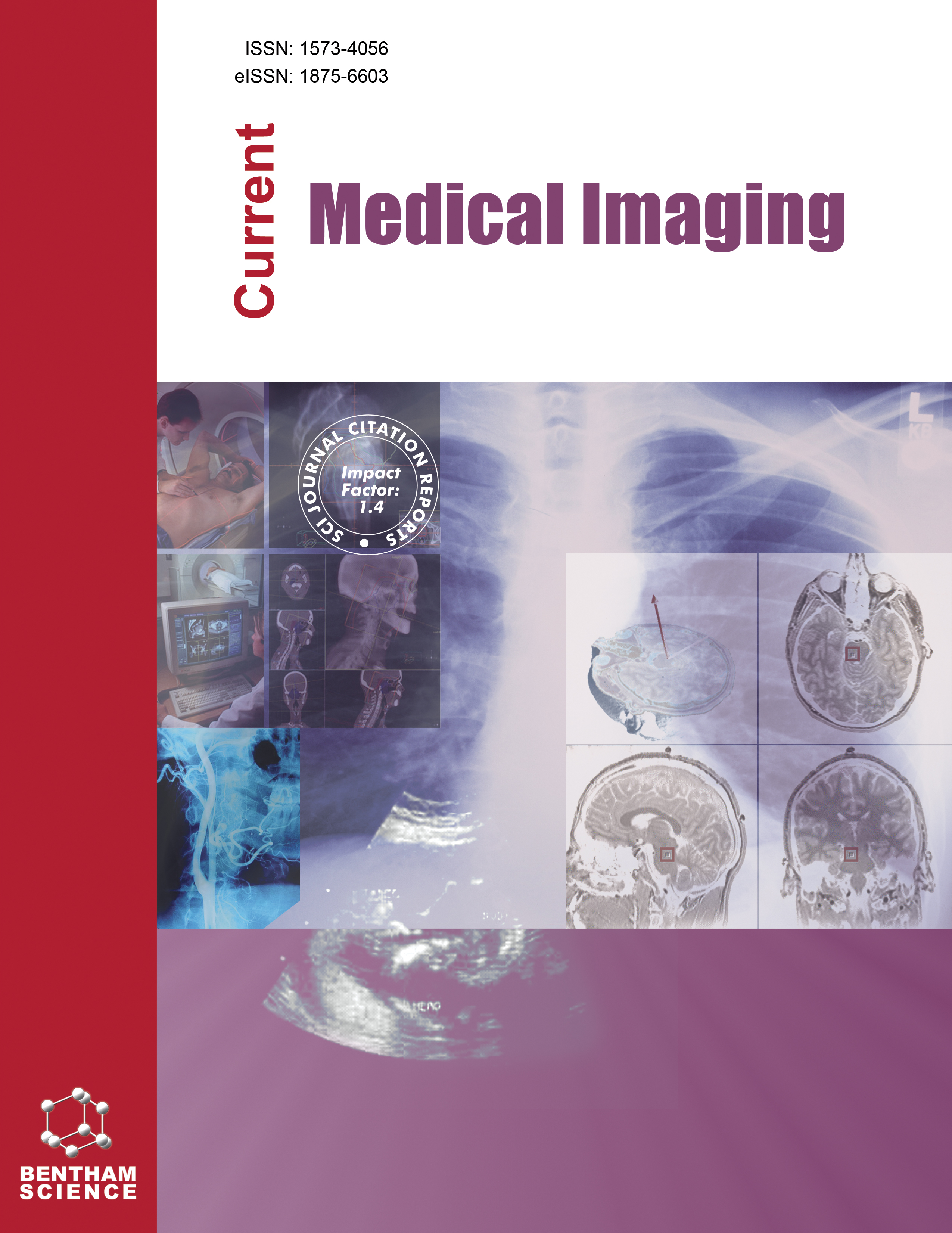
-
oa FedPneu: Federated Learning for Pneumonia Detection across Multiclient Cross-Silo Healthcare Datasets
-
-
- 25 Jun 2024
- 06 Nov 2024
- 02 Jan 2025
Abstract
Pneumonia is an acute respiratory infection that has emerged as the predominant catalyst for escalating mortality rates worldwide. In the pursuit of the prevention and prediction of pneumonia, this work employs the development of an advanced deep-learning model by using a federated learning framework. The deep learning models rely on the utilization of a centralized system for disease prediction on the medical imaging data and pose risks of data breaches and exploitation; however, federated learning is a decentralized architecture which significantly reduces data privacy concerns.
The federated learning works in a distributed architecture by sending a global model to clients rather than sending the data to the model. The proposed federated deep learning-based FedPneu computer-aided diagnosis model has been implemented in 2, 3, 4, and 5 clients architecture for early pneumonia detection using X-ray images. The key parameters configuration include batch size, learning rate, optimizer, decay, momentum, epochs, rounds, and random-split as 32, 0.0001, SGD, 0.000001, 0.9, 10, 100, and 42, respectively.
The results of the proposed federated deep learning-based FedPneu model have been provided in terms of round-wise accuracy, loss, and computational time. The highest accuracy of 85.632% has been achieved with 2-clients federated deep learning architecture, whereas, 3, 4, and 5 clients architecture achieved 85.536%, 76.112%, and 74.123% accuracies, respectively.
In the proposed privacy-protected federated deep learning-based FedPneu model, the two-client architecture has been resulted as the most optimal framework for pneumonia detection among 3-clients, 4-clients, and 5-clients architecture. The model works in a collaborative and privacy-protected framework with a multi-silo dataset which could be highly beneficial for healthcare departments to maintain patient’s data privacy with improved prediction outcomes.