- Home
- Books
- Advanced Technologies for Realizing Sustainable Development Goals: 5G, AI, Big Data, Blockchain, and Industry 4.0 Application
- Chapter
One-Dimensional Convolutional Neural Network for Data Classification
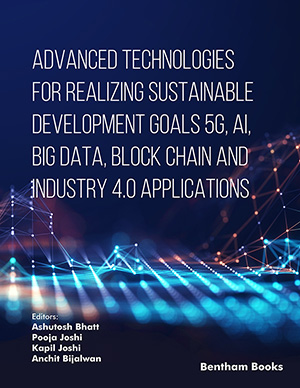
- Authors: Dipankar Dutta1, Soumya Porel2, Debabrata Tah3, Paramartha Dutta4
-
View Affiliations Hide Affiliations1 Department of Computer Science and Engineering, University Institute of Technology, The University of Burdwan, Golapbag (North), Burdwan, PIN 713104, West Bengal, India 2 Department of Artificial Intelligence Software, Qualcomm Inc, Hyderabad, Telangana, India 3 Department of Computer Science and Engineering, Vellore Institute of Technology, Vandalur Road, Rajan Nagar, Chennai, PIN-600127, Tamil Nadu, India 4 Department of Computer and System Sciences, Visva-Bharati University, Santiniketan, PIN731235, West Bengal, India
- Source: Advanced Technologies for Realizing Sustainable Development Goals: 5G, AI, Big Data, Blockchain, and Industry 4.0 Application , pp 37-62
- Publication Date: October 2024
- Language: English
CNN has emerged as the de-facto standard for several machine learning (ML) and computer vision applications. It is known for its classification and feature extraction capabilities. Many ML techniques require separate handcrafted feature extraction steps before classification, which are "sub-optimal" in nature. Unlike these, CNN extracts "optimal" features directly from raw data, enabling it to enhance classification accuracy. Two-dimensional CNN (2D-CNN) is the most common one, where inputs to the CNNs are 2D in nature, such as images. Here, we used 1D-CNN for data classification as we used 1D inputs. 1D-CNN has lower computational complexity than 2D-CNN. Mainly for this, we preferred 1D-CNN over 2D-CNN. To demonstrate the superiority of the proposed generic classifier, we compared its classification accuracies with several other generic classifiers. We used 21 benchmark data sets from the UCI machine learning repository to achieve this. Tests prove the superiority of the proposed 1D-CNN-based generic classifier. Many 1D-CNN-based application-specific classifiers are proposed in the literature, but the proposed classifier is applicable for many types of tabular data i.e., it is a generic classifier.
-
From This Site
/content/books/9789815256680.chapter-3dcterms_subject,pub_keyword-contentType:Journal105
